Purpose: In this
lab, the process of georectifying historic imagery will be addressed, using
Pix4D and ArcMap. The purpose is to gain experience with Pix4D, to understand
the importance of Ground Control Points, and to create a dataset of historical
imagery.
Data Collection: For the lab, historical imagery data from 1939 was
downloaded from the Wisconsin database for historical imagery (figure 1) (https://maps.sco.wisc.edu/WHAIFinder/#12/44.7885/-91.5292).
Each white dot represents a different historical image, attached with the name
of the image and it’s roll number.
 |
Figure 1. The example of where to find data for this lab. Each white dot represents a different image. |
The white dot also represents the geographic center of each
image, which we used to map the approximate location of where the image was
located. To do that, we digitized the dots in ArcMap and created a separate
shapefile, where we also calculated latitude, longitude, altitude, and provided
the image name in the attribute table. Once the attribute table was created, we
exported the table as a dBASE table, then converted it into an XLS file. Once
we converted it into an XLS file, we got ride of certain fields within the
attribute table (i.e. every field except latitude, longitude, and elevation),
then converted the file into a CSV file.
Data Processing:
Once the CSV file was created, we started importing the data into Pix4D. We
first imported the file containing the historical imagery, and then assigned
the CSV file to the historical imagery. Once the CSV file was assigned to the
historical imagery, we created a 3D model that yielded certain rectifications
like a Mosaic, or a Digital Surface Model (DSM).
Results: The results in Pix4D yielded figure 2. The map is of the
of the city limits of Eau Claire, WI, and it seemed to rectify well (i.e. there
wasn’t any trouble placing the right image to the accurate geographical
location). In comparing the mosaic to a basemap of Eau Claire in present day
(figure 3), it seems mostly accurate. The roads on the eastern portion of the
map don’t match up entirely, and the river on the northern//south western
portion of the map are a little off compared where the river is flowed.
However, for manually digitizing the white points and not using precise
location, the images seem to be in their approximate location.
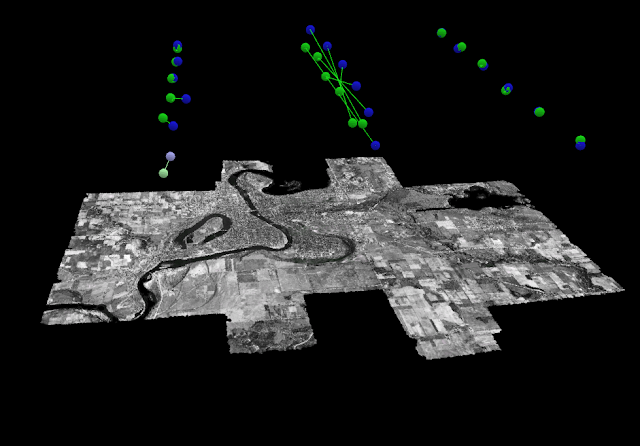 |
Figure 2. The Pix4D result of rectifying the historical imagery. |
In comparing 1939 Eau Claire to
present-day Eau Claire, it seems like urban sprawl moved south after 1939. In
looking at figure 3, it seems like most of the southern portion of the
photograph is farmland; however, in looking at the present-day photograph of
Eau Claire (figure 4), that whole area became residential neighborhoods. One
can also see the emergence of major roads after 1939, with the interstate
spanning the southern portion of figure 4, and Clairemont spanning the center
portion of the photograph. Certain features stayed the same, like most bridges
crossing the river, or most of the neighborhoods in the center of the
photograph.
In comparing the river, it’s hard to tell if there have been
major changes because the mosaic is skewed west compared the present-day map.
The islands in Dell Pond (north part of the photograph) seem similar, and Lake
Altoona (eastern part of the photograph) seems relatively the same.
 |
Figure 3. The historical image of Eau Claire over the current landscape. |
 |
Figure 4. Present day aerial image of Eau Claire. |
Discussion: In
creating the mosaic of the historical imagery, I didn’t have any trouble in
doing the assignment. I thought my files rendered well and produced a
relatively accurate image (that with a little georeferencing, could be even
more accurate), and I found the process smooth and painless.
This lab is important because we produced a valuable dataset
with mosaicking historical imagery. Historical imagery could be used to map
urban sprawl, or to see how certain water features have changed over time.